Partly continuing on from my previous post…
So I think we’d all agree that applied mathematics is a venerable field of its own. But are you tired of hearing statistics distinguished from “data science“? Trying to figure out the boundaries between data science, statistics, and machine learning? What skills are needed by the people in this field (these fields?), do they also need domain expertise, and are there too many posers?
Or are you now confused about what is statistics in the first place? (Excellent article by Brown and Kass, with excellent discussion and rejoinder — deserving of its own blog post soon!)
Or perhaps you are psyched for the growth of even more of these similar-sounding fields? I’ve recently started hearing people proclaim themselves experts in info-metrics and uncertainty quantification. [Edit: here’s yet another one: cognitive informatics.]
Is there a benefit to having so many names and traditions for what should, essentially, be the same thing, if it hadn’t been historically rediscovered independently in different fields? Is it just a matter of branding, or do you think all of these really are distinct specialties?
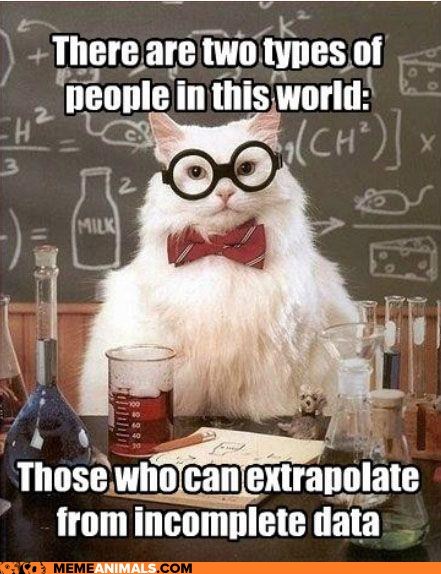
Given the position in my last post, I might argue that you should complete Chemistry Cat’s sentence with “…and those who can quantify their uncertainty about those extrapolations.” And maybe some fields have more sophisticated traditions for tackling the first part, but statisticians are especially focused on the second.
In other words, much of a statistician’s special unique contribution (what we think about more than might an applied mathematician, data scientist, haruspicer, etc.) is our focus on the uncertainty-related properties of our estimators. We are the first to ask: what’s your estimator’s bias and variance? Is it robust to data that doesn’t meet your assumptions? If your data were sampled again from scratch, or if you ran your experiment again, what’s the range of answers you’d expect to see? These questions are front and center in statistical training, whereas in, say, the Stanford machine learning class handouts, they often come in at the end as an afterthought.
So my impression is that other fields are at higher risk of modeling just the mean and stopping there (not also telling you what range of data you may see outside the mean), or overfitting to the training data and stopping there (not telling you how much your mean-predictions rely on what you saw in this particular dataset). On the other hand, perhaps traditional stats models for the mean/average/typical trend are less sophisticated than those in other communities. When statisticians limit our education to the kind of models where it’s easy to derive MSEs and compare them analytically, we miss out on the chance to contribute to the development & improvement of many other interesting approaches.
So: if you call yourself a statistician, don’t hesitate to talk with people who have a different title on their business cards, and see if your special view on the world can contribute to their work. And if you’re one of these others, don’t forget to put on your statistician hat once in a while and think deeply about the variability in the data or in your methods’ performance.
PS — I don’t mean to be adversarial here. Of course a good statistician, a good applied mathematician, a good data scientist, and presumably even a good infometrician(?) ought to have much of the same skillset and worldview. But given that people can be trained in different departments, I’m just hoping to articulate what might be gained or lost by studying Statistics rather than the other fields.